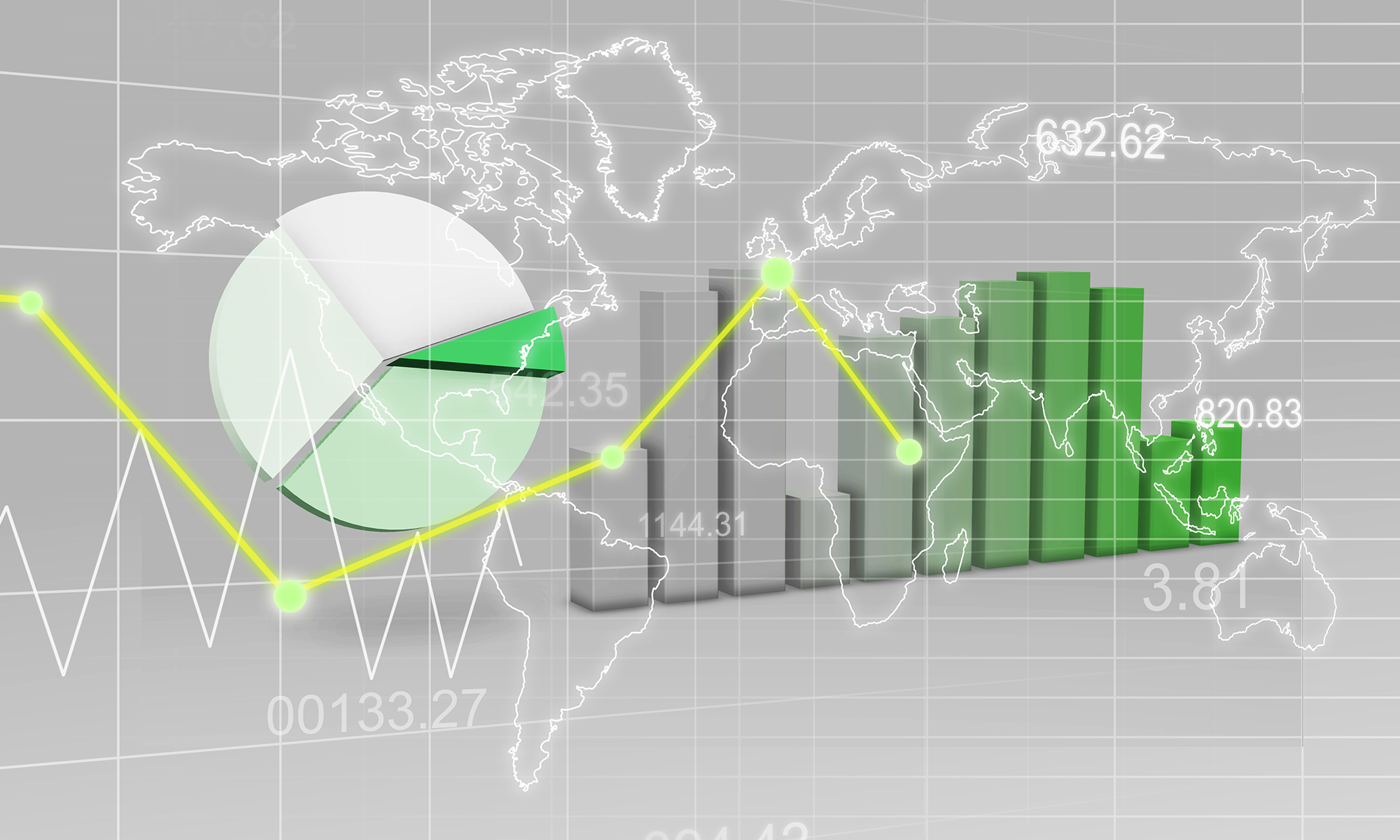
About the Journal
This journal is centrally concerned with the complex problem of estimating the past, current, and future market value of residential and commercial real estate. Furthermore, valuation techniques developed for real estate can often be applied to other assets. We expect the methods presented to apply to valuing houses, office buildings, and equipment such as standard machinery and robots. Real estate has the primary focus simply because of the vast amount of available data and the fact that measured and unmeasured features influence real estate market values. In particular, unmeasured features such as aesthetics, condition, quality, and view make accurate valuation particularly difficult.